Jess Hall
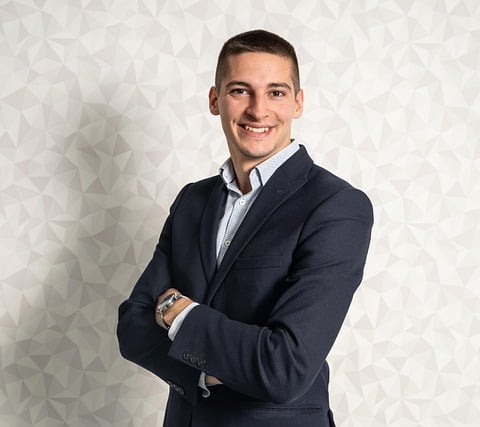
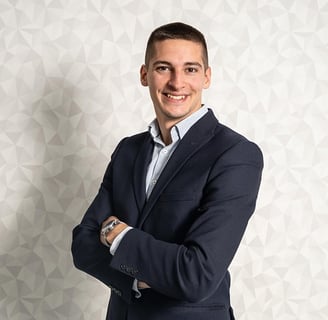
Professional Summary:
Jess Hall is a dynamic professional in the field of AI-driven personalized recommendation, specializing in using artificial intelligence to analyze user behavior and deliver tailored product and content recommendations. With a strong background in data science, machine learning, and user experience design, Jess is dedicated to enhancing customer engagement and satisfaction by creating intelligent, data-driven recommendation systems. Her work empowers businesses to deliver personalized experiences that drive conversions, loyalty, and long-term growth.
Key Competencies:
AI-Powered Recommendation Systems:
Develops advanced AI algorithms to analyze user behavior, preferences, and interactions, generating highly relevant product and content recommendations.
Utilizes machine learning models, including collaborative filtering, content-based filtering, and hybrid approaches, to optimize recommendation accuracy.
User Behavior Analytics:
Proficient in integrating and analyzing data from multiple sources, including browsing history, purchase patterns, and social interactions, to uncover actionable insights.
Builds scalable data pipelines to process and interpret large datasets for real-time recommendations.
Personalization Strategies:
Designs AI-driven frameworks to deliver personalized experiences across various platforms, such as e-commerce websites, streaming services, and mobile apps.
Implements A/B testing and continuous learning mechanisms to refine recommendation models and improve user engagement.
Machine Learning Expertise:
Builds and optimizes machine learning models for recommendation systems, including natural language processing (NLP) for content understanding and reinforcement learning for dynamic adaptation.
Stays updated with the latest AI advancements to drive innovation in personalized recommendation technologies.
Cross-Functional Collaboration:
Collaborates with product managers, marketing teams, and business leaders to align recommendation strategies with organizational goals.
Provides training and support to ensure the successful adoption of AI tools.
Career Highlights:
Developed an AI-powered recommendation engine that increased conversion rates by 20% for a leading e-commerce platform.
Designed a personalized content recommendation system that boosted user engagement by 25% for a global media company.
Published influential research on AI applications in personalized recommendation, earning recognition at international tech conferences.
Personal Statement:
"I am passionate about leveraging AI to create personalized experiences that delight users and drive business success. My mission is to build intelligent recommendation systems that understand user needs, anticipate preferences, and deliver meaningful value."
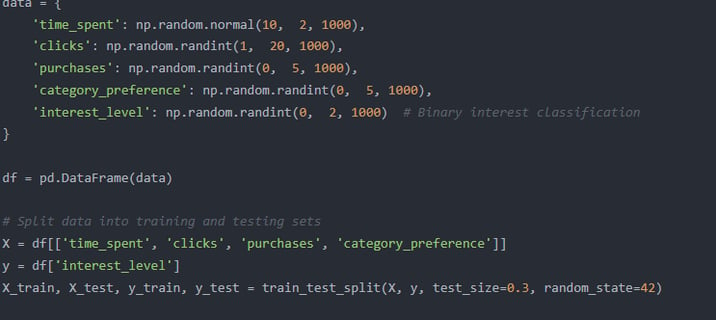
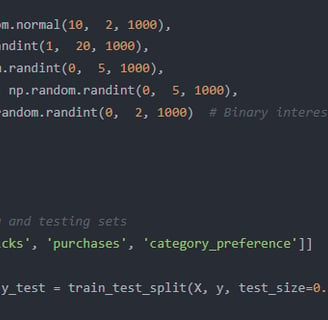
Fine-Tuning Necessity
Fine-tuning GPT-4 is essential for this research because publicly available GPT-3.5 lacks the specialized capabilities required for analyzing complex user behavior data and generating highly personalized recommendations. Personalized recommendation systems involve highly domain-specific knowledge, intricate user interaction patterns, and nuanced preference predictions that general-purpose models like GPT-3.5 cannot adequately address. Fine-tuning GPT-4 allows the model to learn from personalized recommendation datasets, adapt to the unique challenges of the domain, and provide more accurate and actionable insights. This level of customization is critical for advancing AI’s role in personalized recommendation and ensuring its practical utility in real-world scenarios.
Past Research
To better understand the context of this submission, I recommend reviewing my previous work on the application of AI in personalized recommendation, particularly the study titled "Enhancing Personalized Recommendations Using Machine Learning Models." This research explored the use of collaborative filtering and deep learning techniques for improving recommendation accuracy. Additionally, my paper "Adapting Large Language Models for Domain-Specific Applications in Personalized Recommendation" provides insights into the fine-tuning process and its potential to enhance model performance in specialized fields.